
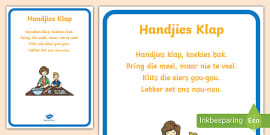
The SNE foundation is the modeling of pairwise similarities by transforming Euclidean distances into likelihoods of selecting neighbors and, being centered on a probabilistic model, it uses different bi-dimensional spaces and combines them into a single model of similarity, therefore leading to a good visualization of data. More recent methods, such as the stochastic neighbor embedding (SNE) or the local linear embedding (LLE), aim to represent the similarity structure of objects by involving a two-dimensional visualization, where the higher the similarity between pairs, the less the distance between them. Therefore, they may not provide a good visualization of data. Both techniques also embed a cost function more reliable with the modeling of large dissimilarities rather than the small ones. Dimensionality reduction can be performed with different kinds of procedures : Classical methods like the metric multi-dimensional scaling (MDS) and the principal components analysis are fast and efficient but they may fail to identify the real structure of datasets when they contain a nonlinear configuration. Moreover, the dropping of uninformative attributes may help to highlight the best predictors and to improve the model’s accuracy. The data reduction, indeed, allows the decrease of the storage amount and that of computational time, an easier understanding of data distribution, the improvement of visualization, classification and clusterization of high dimensional data.

Still, despite the high-dimensionality, because of the redundancy and multicollinearity of variables, data can be reduced and represented by fewer features. These can be daunting to be analyzed and may fail to satisfy the assumptions required by common statistical models. Recent technological innovations in many areas of animal behavioral research, allow the collection of huge, complex, and often high-dimensional data sets. Our results indicated that the t-distributed stochastic neighbor embedding (t-SNE), successfully been employed in several studies, showed a good performance also in the analysis of indris’ repertoire and may open new perspectives towards the achievement of shared methodical techniques for the comparison of animal vocal repertoires. The t-distributed stochastic neighbor embedding (t-SNE) mapping indicated the presence of eight different groups, consistent with the acoustic structure of the a priori identification of calls, while the cluster analysis revealed that an overlay between distinct call types might exist. We submitted the set of acoustic features first to a t-distributed stochastic neighbor embedding algorithm, then to a hard-clustering procedure using a k-means algorithm.
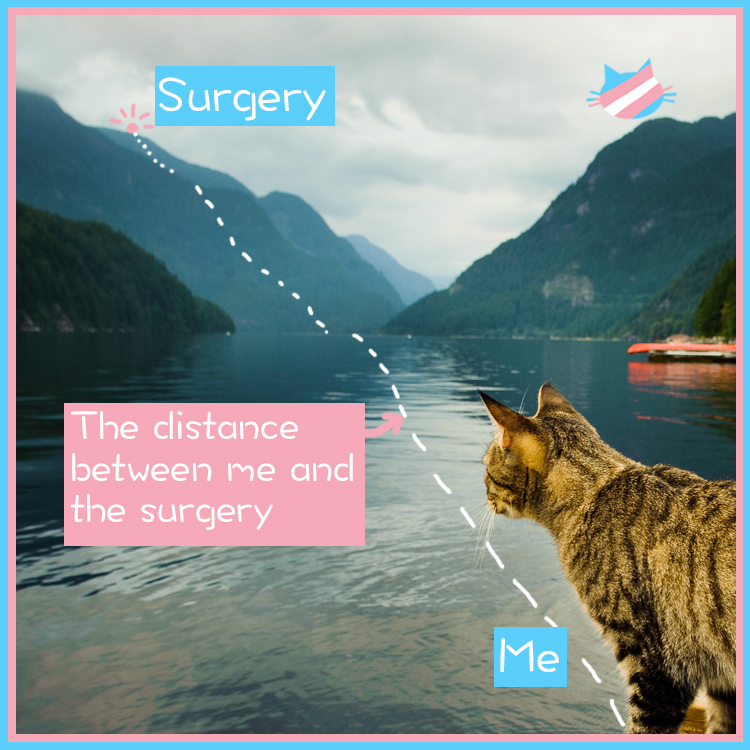
We split each sound into ten portions of equal length and, from each portion we extracted spectral coefficients, considering frequency values up to 15,000 Hz. Here, we introduced a computational method used to examine 3360 calls recorded from wild indris ( Indri indri) from 2005–2018. Although there is a growing number of researches focusing on acoustic communication, the lack of shared analytic approaches leads to inconsistency among studies.
